CTN-0094: Individual Level Predictive Modeling of Opioid Use Disorder Treatment Outcome
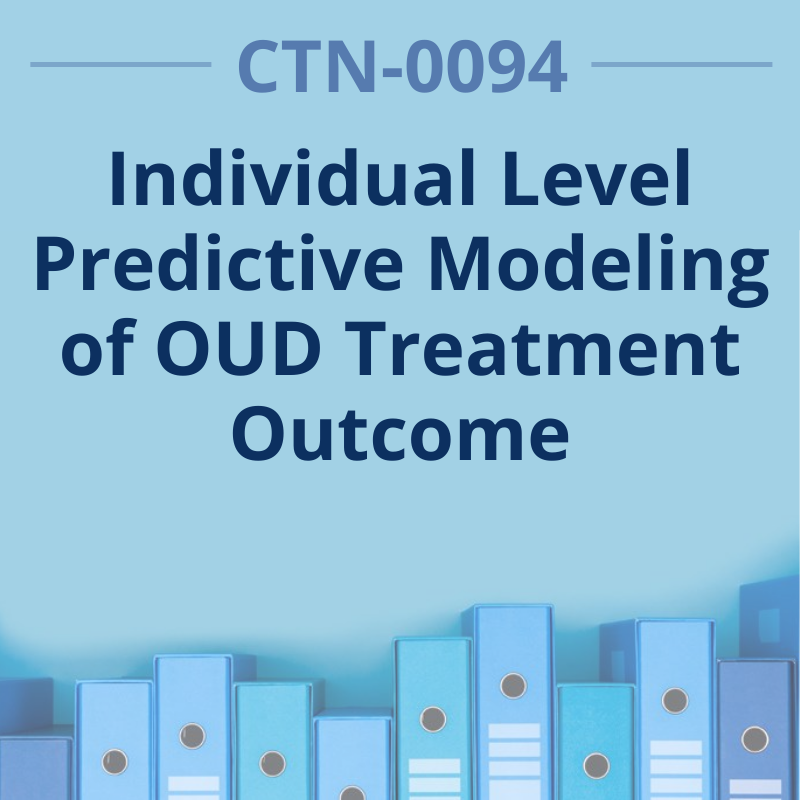
Sean Luo, MD, PhD
Lead Investigator
Columbia University
Department of Psychiatry
Sxl2101@columbia.edu
Daniel J. Feaster, PhD
Lead Investigator
University of Miami Miller School of Medicine
Center for Family Studies
dfeaster@miami.edu
A persistent problem in the dissemination of medication treatments for opioid use disorder (MOUD) is patient dropout, and matching patients to suitable medication early has the potential to minimize dropout. The overall objective of this secondary data analysis study is to develop and disseminate individual level risk prediction models using harmonized data collected from three multi-site clinical trials from the CTN, in order to predict specific clinical outcomes (drop out; relapse) for patients treated with MOUD, including methadone, buprenorphine, or extended-release depot naltrexone. The relative importance of predictors in the best predictive models will be estimated, which may facilitate refinement of common data elements for future OUD studies. The comprehensive, harmonized database of treatment data created in this study can be used for future secondary data analysis studies and will provide a replicable data pipeline to process and validate OUD data in future protocols.
Funded by the NIH HEAL InitiativeSM.
Related Resources
Node Involvement
Lead Node(s):
All Participating Nodes: