CTN-0111: COVID-19 and Substance Misuse Case Identification Using Data Science: A Retrospective Cohort Study
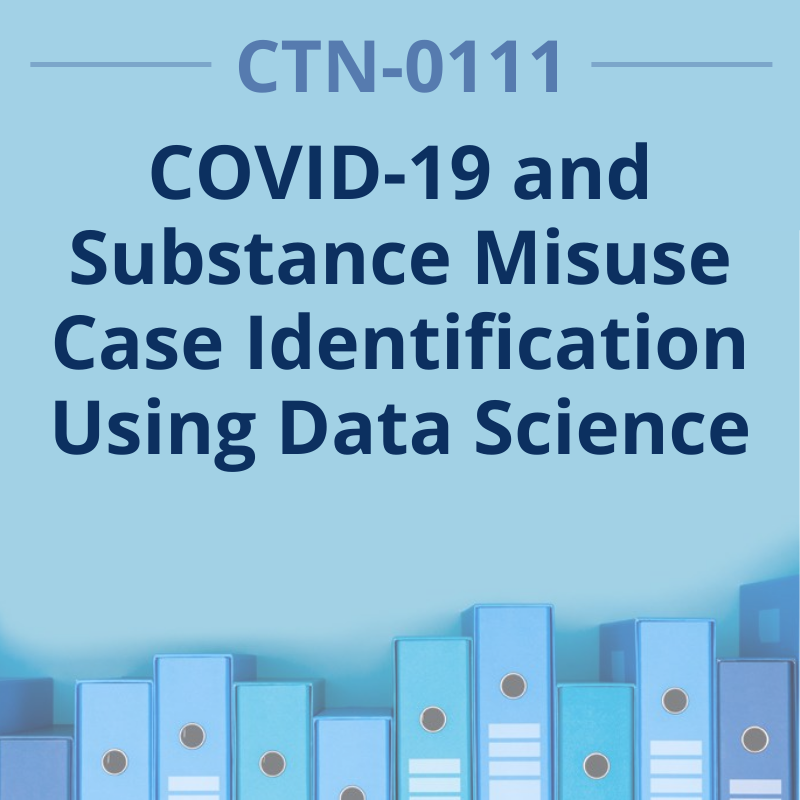
Niranjan Karnik, MD, PhD
Lead Investigator
Rush University, Department of Psychiatry & Behavioral Sciences
niranjan_karnik@rush.edu
This project will assess a potentially important tool set empowered by artificial intelligence techniques for detecting and classifying substance misuse and COVID-19 from the electronic health record (EHR). Specificity and sensitivity of this digital classifier would be thoroughly evaluated using real world EHRs derived from 60,000 patients who have been screened for COVID-19. This research will serve as the first step towards a universal screener that leverages available data in the EHR for opioid misuse prevention and treatment.
Primary Findings
Topic modeling yielded 10 topics per subgroup and highlighted unique comorbidities associated with Unhealthy Opioid Use (UOU) and COVID-19 (e.g., HIV) and no UOU and COVID-19 (e.g., diabetes). In the regression analysis, each incremental increase in the classifier’s predicted probability of UOU was associated with 1.16 higher odds of COVID-19 outcome severity (odds ratio 1.16, 95% CI 1.04-1.29; P=.009).

Primary Outcomes Article: Thompson HM, et al. Machine Learning Techniques to Explore Clinical Presentations of COVID-19 Disease Severity and to Test the Association with Unhealthy Opioid Use (UOU): Retrospective Cross-Sectional Cohort Study. JMIR Public Health and Surveillance 2022;8(12):e38158. [get article]
Related Resources
Node Involvement
Lead Node(s):
All Participating Nodes: